TUTORIALS

Elisabeth LEX
Graz University of Technology, Austria
Psychology-informed Recommender Systems
Assoc.-Prof. Dr. Elisabeth Lex is a tenured associate professor of Applied Computer Science at Graz University of Technology (TUG) and PI of the Social Computing Lab at TUG. Her habilitation is on "Modeling and Predicting User Behavior in Web-based Systems". Elisabeth is co-PI of the transuniversity research project "Polarization in Public Opinion". She was work package leader in the FP7 IP Learning Layers, in which she researched cognition-inspired recommender systems and scientific coordinator of the Marie Curie IRSES Web Information Quality Evaluation Initiative (WIQ-EI) project, where she worked in quantifying information quality and credibility aspects of social media. She was task leader in the H2020 Analytics for Everyday Learning (AFEL) project, in which she researched psychology-informed recommender systems and opinion formation in online collaboration networks. Elisabeth was a member of the Expert Group on Altmetrics, which advised the European Commission, DG Research and Innovation. The expert group developed policies for the commission on how to use altmetrics to assess the impact of scientific artefacts. Elisabeth has published more than 100 scientific publications in venues such as WWW (TheWebConf), ACM Conference on Hypertext and Social Media (HT), ACM Conference on Recommender Systems, ACM UMAP, ECIR, ISMIR, as well as in journals such as UMUAI, EPJ Data Science, Frontiers in AI, Transactions of the International Society for Music Information Retrieval (TISMIR), or the International Journal of Human–Computer Interaction. Elisabeth regularly gives invited talks about her research and acts as Senior PC member, PC member and co-organizer and co-chair at venues such as ACM IUI, ACM RecSys, ACM Web Science or ACM HT. Among other courses at Graz University of Technology, Elisabeth teaches Computational Social Systems, Advanced Information Retrieval, Human Rights and Artificial Intelligence, and Scientific Writing.
-
Personalized recommender systems have become critical means to support human decision making in today's online world. Most of today's recommender systems are data-driven and exploit behavioral data to learn user models and to predict user preferences. While such systems can produce useful recommendations, they do not incorporate the underlying psychological reasons for user behavior in the algorithms' design. The aim of this tutorial is to present recent work in psychology-informed
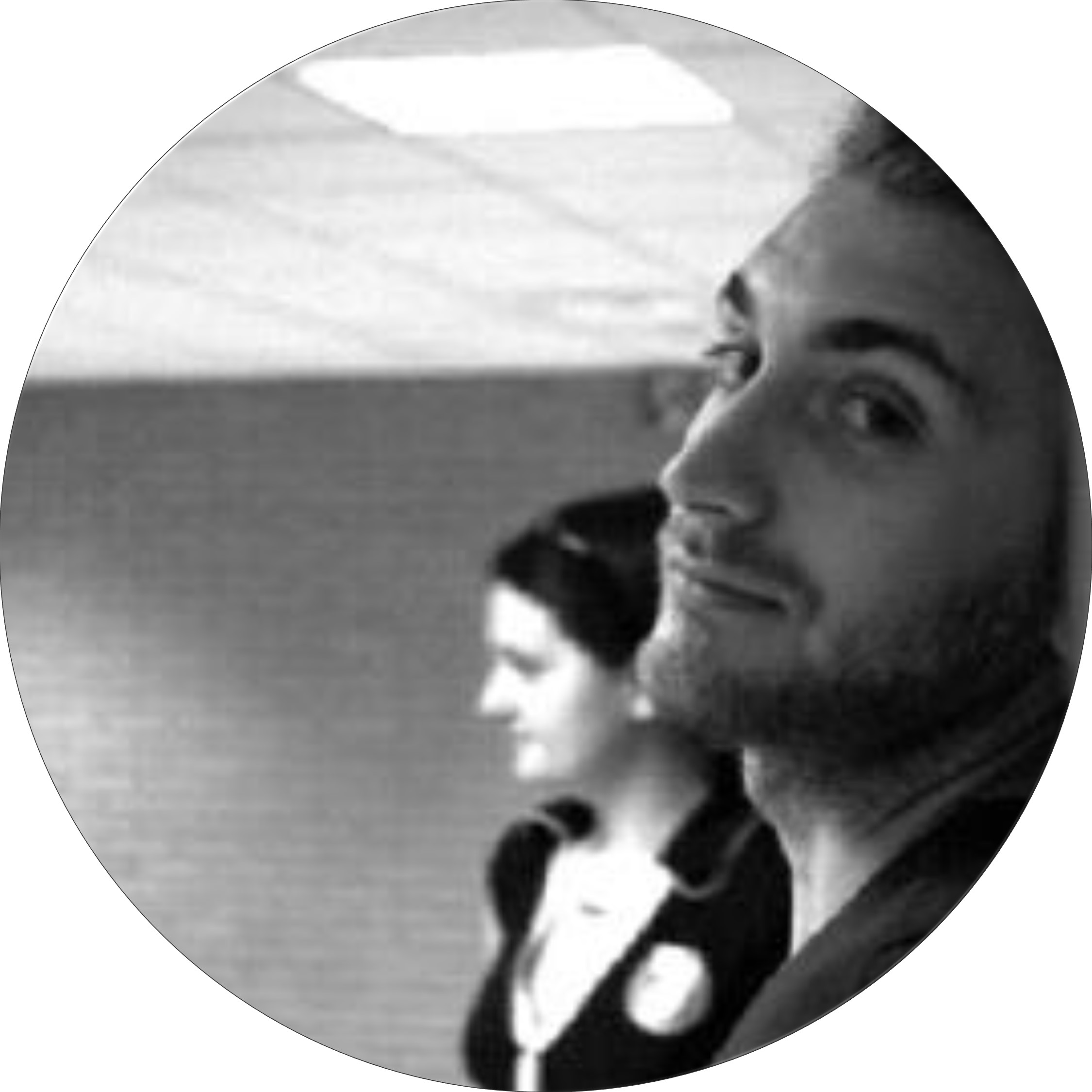
Giovanni PETRI
ISI Foundation, Italy
A crash-course in TDA and higher-order dynamics
I am a Senior Research Scientist at ISI Foundation working on topological approaches to complex networks and their underlying geometry, with special attention to the topology of brain structure and dynamics. Interests: Topology and Predictability of Complex Systems, Computational Neuroscience, Cognitive Control. Education: PhD in Complex Networks, 2012, Imperial College London; MSc in Theoretical Physics, 2008, University of Pisa; BSc in Physics, 2005, University of Pisa
-
The tutorial will provide a primer and overview of recent advances in topological data analysis and higher-order models. The first part will focus on the basics of persistent homology and Mapper, with examples of applications to datasets, and on some tools to reconstruct higher order structures from lower dimensional projects. The second part will focus on the dynamical models with interactions beyond pairwise, with direct examples of spreading, synchronization and consensus. Both parts will feature a theoretical introduction and hands-on exercises.
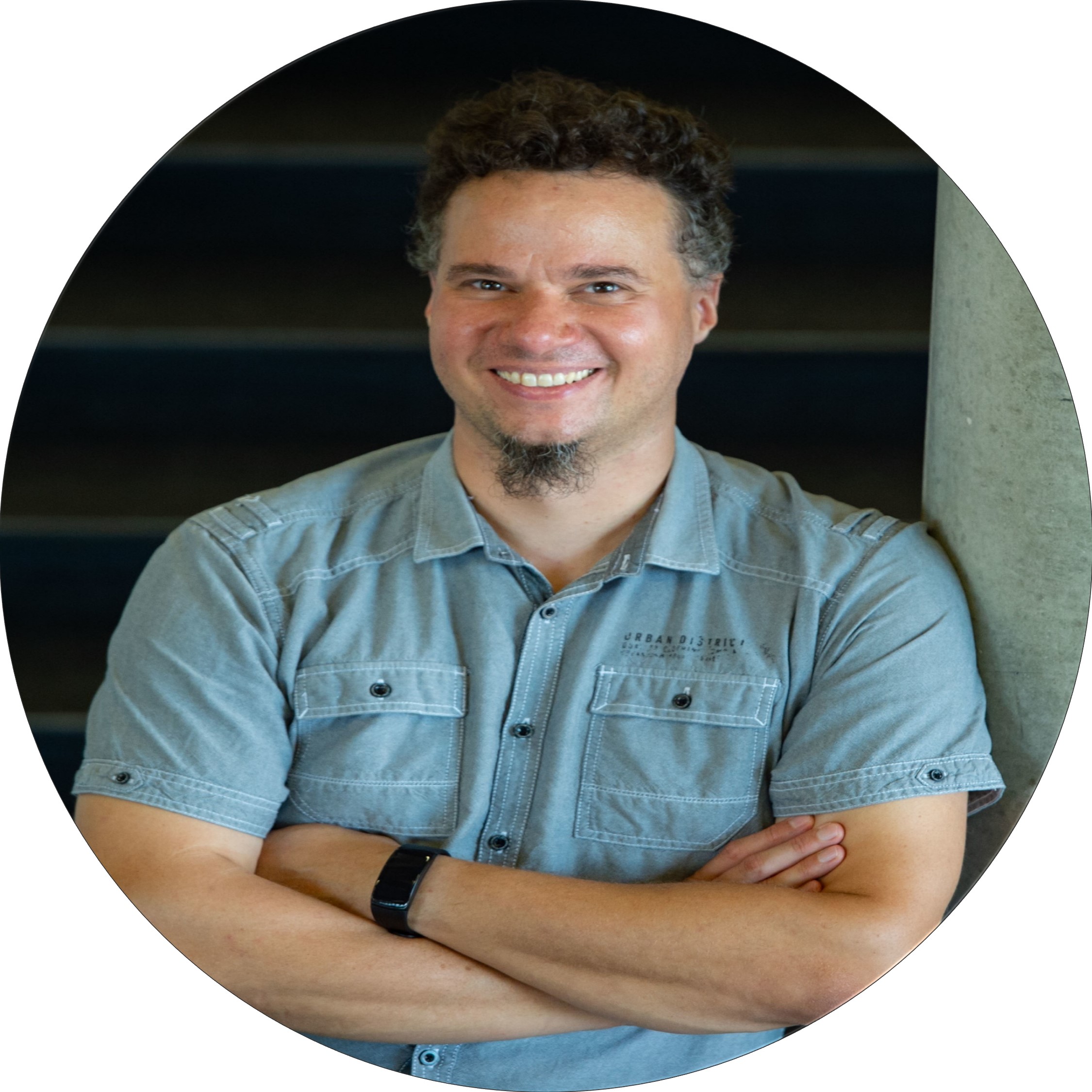
Markus SCHEDL
Johannes Kepler University, Austria
Psychology-informed Recommender Systems
Markus Schedl is a Full Professor at Johannes Kepler University (JKU) Linz, Austria, affiliated with the Institute of Computational Perception, where he leads the group Multimedia Mining and Search (MMS). In addition, he is head of the Human-centered AI group at the Linz Institute of Technology (LIT) AI Lab. His areas of expertise include recommender systems, user modeling, information retrieval, machine learning, natural language processing, multimedia, data analysis, and web mining.
He (co-)authored more than 200 refereed conference papers and journal articles, among others, published in ACM Multimedia, RecSys, ICMR, SIGIR, ECIR, ISMIR, IEEE Visualization; Journal of Machine Learning Research, ACM Transactions on Information Systems, IEEE Transactions on Affective Computing, IEEE Multimedia, User Modeling and User-Adapted Interaction (UMUAI), and PLOS ONE. Furthermore, he is associate editor of the Springer International Journal of Multimedia Information Retrieval and the Transactions of the International Society for Music Information Retrieval. He served as a program co-chair for the International Society for Music Information Retrieval (ISMIR) conference in 2020, and on the program committee of other top-tier conferences, including WEB (formerly WWW), RecSys, ICMR, and ACM Multimedia (as area chair).
He has been leading and co-leading projects funded by the Austrian Science Fund (FWF), the Austrian Research Promotion Agency (FFG), and the European Commission (EC). He also maintain collaborations with industry, for instance with Siemens, Spotify, and Deezer. Furthermore, he serves as consultant on the topics mentioned above.
-
Personalized recommender systems have become critical means to support human decision making in today's online world. Most of today's recommender systems are data-driven and exploit behavioral data to learn user models and to predict user preferences. While such systems can produce useful recommendations, they do not incorporate the underlying psychological reasons for user behavior in the algorithms' design. The aim of this tutorial is to present recent work in psychology-informed
Recent Comments